Frontiers in AI
Access to content: https://digital.ecai2020.eu/frontiers-in-ai-2/
Description of the event:
This track consists of four sessions where four selected invited speakers will be presenting talks to make the AI community aware of particularly interesting new research results, directions, and trends.
Frontier in AI talks should appeal to the broad AI community, are of free to attend and will run in parallel with other sessions in the last slot in the morning (11:45-13:00 CEST):
Monday, August 31, 11:45 CEST: BLAI BONET: “Representation learning and synthesis for generalized planning”.
Recent work in planning and learning is concerned with the task of inferring general plans from either models or data that solve multiple problems from the same domain (e.g., any blocks world problem). In this talk, I will address generalized planning from a model-based perspective conveying the progress made and challenges ahead. We will see how multiple problem instances can be captured with a finite but non-deterministic abstraction based on qualitative numerical planning (QNP) that can be solved using off-the-shelf (FOND) planners. QNP problems that involve numerical and boolean features can be used to capture multiple instances of a planning problem while avoiding undecidability issues. The QNP abstraction can be either learned from samples and a first-order domain model, or directly learned from images (pixels) with the help of deep neural nets.
Tuesday, September 1, 11:45 CEST: RADA MIHALCEA: “People-Centric Language Computing”
Language is not only about the words, it is also about the people. While much of the work in computational linguistics has focused almost exclusively on words (and their relations), recent research in the emerging field of computational sociolinguistics has shown that we can effectively leverage the close interplay between language and people. In this talk, I will explore this interaction, and show (1) that we can develop cross-cultural language models to identify words that are used in significantly different ways by speakers from different cultures; and (2) that we can effectively use information about the people behind the words to build better language representations.
Wednesday, September 2, 11:45 CEST: MAGDALENA ORTIZ: “Knowledge and Reasoning for Intelligent Systems”.
Since the early days of AI, Knowledge Representation and Reasoning (KR) has pursued the goal of capturing human knowledge in forms that can be stored in machines and used for automated inference, enabling computers to draw conclusions analogous to the ones we humans draw from our own knowledge. Although some goals of the field’s original research agenda proved elusive, KR has delivered solutions to many central AI problems. In this talk, we will discuss a few selected areas where KR has proved very successful, if not necessarily at replicating the whole spectrum of human intelligence, certainly at achieving more intelligent information systems. For example, explicit knowledge captured in ontologies is a powerful tool to access information on the Web, and for facilitating the management of data that may be unstructured, heterogeneous, and incomplete. Knowledge captured in rule languages can be leveraged for solving combinatorial problems like configuration, diagnosis, and planning. Beyond these success stories, we will also touch on some current AI challenges where we believe KR can play a central role: can explicit knowledge and automated reasoning help us make modern AI more transparent, safer, and more trustworthy?
Thursday, September 3, 11:45 CEST: RANDY GOEBEL: “At the frontier of AI Application: balancing technology push and application pull”.
The pace at which AI theory is being delivered to application has accelerated in the last decade, creating some impressive value in some areas (e.g., health and legal informatics, manufacturing, supply chain management), but raising warning flags about trust and ethics. Both the promise and the challenges are evident in the application of AI to automotives and automated autonomous systems, especially in the choice of technologies, tradeoffs in where intelligence is required (i.e., the autonomous system or the infrastructure), and the emerging role of explainable AI, both in improving the transparency, trust, and robustness of systems, and in informing social systems and regulators about how to confirm their safety. We try to highlight salient aspects of these challenges, and provide some context for helping to manage the translation of AI theory to application.
This track is sponsored by IOS Press.
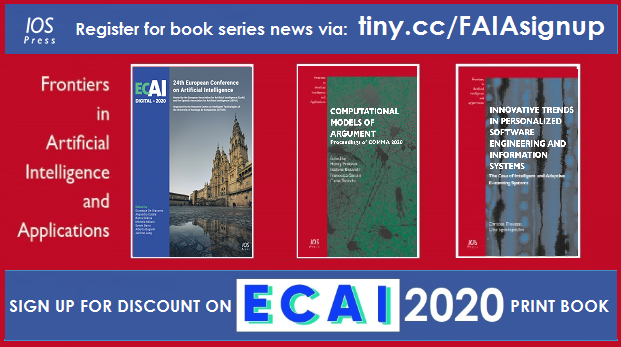