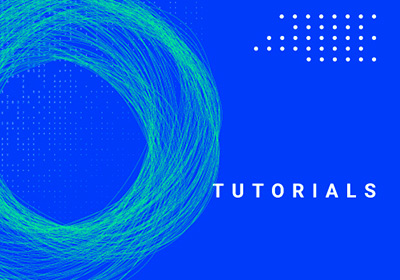
Data Driven Policy Learning in Real World Multi-Agent Environments
August 30, 10:45 am - 12:15 pm (CEST)
Speakers: Varuna De Silva
Tutorial website: https://sites.google.com/view/varunadesilva/ecai-2020-tutorial-ma-rl?authuser=0
Agenda: Data Driven Policy Learning in Real World Multi-Agent Environments
A fundamental goal of AI is to produce intelligent agents (IAs) that interact with its environment to learn optimal behaviours for autonomous decision making, technically defined as policy learning. Most developments in policy learning algorithms in the past decade have been framed as a Reinforcement Learning (RL) of a single agent, where modelling and predicting behaviour of other agents in the environment is largely unnecessary. Furthermore, the developments are often demonstrated in settings with well-defined utility functions, such as board/video games. However, if AI is to live up to its science-fictional promises to support humanity or even supersede human intelligence, recent algorithmic developments should be scaled to perform in real-world environments where multiple agents interact to accomplish a task. Policy learning in real-world multi-agent environments is extremely complex due to two reasons: (1) The actions of multiple intelligent agents cause the environment to become non-stationary from the perspective of an individual agent. Hence, traditional RL algorithms are not well suited for multi-agent domains. (2) The context of real-world environments is often represented with multiple high-dimensional modalities. In comparison to single agent domains, multi-agent policy learning algorithms suffer from the effects of curse of dimensionality, and high-dimensional context representation further exacerbates this issue. In addition, lack of meaningful data-sets is also partly culpable for comparatively modest progress in the area of multi-agent policy learning. Driverless vehicles (DV), military combat and disaster recovery robots, artificial trading in financial markets, and autonomous communication and language discovery are few ambitious aspirations, where policy learning in multi-agent domains is essential.
Starting with an intuitive explanation of the theoretical underpinnings, this tutorial will present key developments in the area of multi-agent policy learning: namely Multi-agent reinforcement learning and multi-agent imitation learning, which are emerging as key techniques to address the problem of multi agent policy learning. The tutorial will relate to emerging applications of multi-agent policy learning such as driverless vehicle control, sports analytics, urban planning and autonomous generation of video game content. While relating to the real-world applications the tutorial will gently introduce recent attempts at addressing key challenges relate to multi-agent policy learning, such as non-stationarity, communication, selective attention, curriculum learning and generative adversarial policy learning. Furthermore, the discussion will involve, the difference between coordinated learning which is suitable for cooperating agents and learning in the environments where agents compete with each other such as in sports. Tutorial will also introduce the audience to available data sources and experimental platforms to experiment with multi-agent policy learning. Finally, the tutorial will conclude with a discussion on challenges to move theoretical results in the real world applications where agents are required to learn from limited experience.
Varuna De Silva, Senior Lecturer in machine intelligence at Loughborough University.