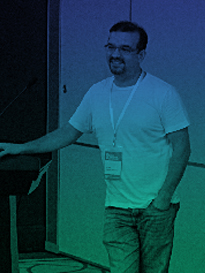
Representation learning and synthesis for generalized planning
Recent work in planning and learning is concerned with the task of inferring general plans from either models or data that solve multiple problems from the same domain (e.g., any blocks world problem). In this talk, I will address generalized planning from a model-based perspective conveying the progress made and challenges ahead. We will see how multiple problem instances can be captured with a finite but non-deterministic abstraction based on qualitative numerical planning (QNP) that can be solved using off-the-shelf (FOND) planners. QNP problems that involve numerical and boolean features can be used to capture multiple instances of a planning problem while avoiding undecidability issues. The QNP abstraction can be either learned from samples and a first-order domain model, or directly learned from images (pixels) with the help of deep neural nets.