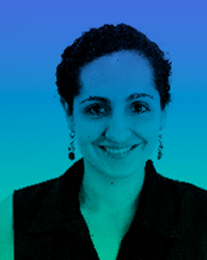
Knowledge and Reasoning for Intelligent Systems
Since the early days of AI, Knowledge Representation and Reasoning (KR) has pursued the goal of capturing human knowledge in forms that can be stored in machines and used for automated inference, enabling computers to draw conclusions analogous to the ones we humans draw from our own knowledge. Although some goals of the field’s original research agenda proved elusive, KR has delivered solutions to many central AI problems. In this talk, we will discuss a few selected areas where KR has proved very successful, if not necessarily at replicating the whole spectrum of human intelligence, certainly at achieving more intelligent information systems. For example, explicit knowledge captured in ontologies is a powerful tool to access information on the Web, and for facilitating the management of data that may be unstructured, heterogeneous, and incomplete. Knowledge captured in rule languages can be leveraged for solving combinatorial problems like configuration, diagnosis, and planning.
Beyond these success stories, we will also touch on some current AI challenges where we believe KR can play a central role: can explicit knowledge and automated reasoning help us make modern AI more transparent, safer, and more trustworthy?